Difference between revisions of "Research"
From Bioinformatics Lab
Line 1: | Line 1: | ||
=='''Research Summary'''== | =='''Research Summary'''== | ||
+ | {{#widget:AddHtml|content=<div style="float:right;margin:20px 40px;"> <img src="cloud.jpg" alt="Word Cloud from Abstraction below publications" /></div>}} | ||
The ultimate goal of biological research is to manipulate traits that are important for medicine, agriculture, and bio-industry. This challenging task first requires good understanding of association between genotype and phenotype. Because of high complexity of genotype as well as phenotype, complexity of the genotype-phenotype association could be even untouchable by combinatorial explosion of the number of possible associations. Therefore, modern genetics needs to be more systematic and predictive. Recently we proposed network-guided approach for genetics of complex traits. First, we construct probabilistic functional gene networks for cells or organisms by benchmarking and integrating heterogeneous multi-omics data that are in general publicly available. Then, using guilt-by-association, and other algorithms of network propagation of known biological information, we predict gene functions, phenotypic effect of loss-of-function, and epistatic interaction. The information can contribute to reconstruction of map between genotype and phenotype. The network-guided genetics method has been effectively applied for various organisms; from simple microbe yeast, to multicellular animal C. elegans, to the reference plant Arabidopsis, to the reference crop rice, and to the human. | The ultimate goal of biological research is to manipulate traits that are important for medicine, agriculture, and bio-industry. This challenging task first requires good understanding of association between genotype and phenotype. Because of high complexity of genotype as well as phenotype, complexity of the genotype-phenotype association could be even untouchable by combinatorial explosion of the number of possible associations. Therefore, modern genetics needs to be more systematic and predictive. Recently we proposed network-guided approach for genetics of complex traits. First, we construct probabilistic functional gene networks for cells or organisms by benchmarking and integrating heterogeneous multi-omics data that are in general publicly available. Then, using guilt-by-association, and other algorithms of network propagation of known biological information, we predict gene functions, phenotypic effect of loss-of-function, and epistatic interaction. The information can contribute to reconstruction of map between genotype and phenotype. The network-guided genetics method has been effectively applied for various organisms; from simple microbe yeast, to multicellular animal C. elegans, to the reference plant Arabidopsis, to the reference crop rice, and to the human. | ||
− | |||
− | |||
− | |||
=='''Research Highlight'''== | =='''Research Highlight'''== | ||
*[[media:research_highlight_004.pdf|2011 Nature Reviews Genetics, Research highlight (Predicting genetic interaction)]] | *[[media:research_highlight_004.pdf|2011 Nature Reviews Genetics, Research highlight (Predicting genetic interaction)]] |
Revision as of 11:55, 1 October 2014
Research Summary
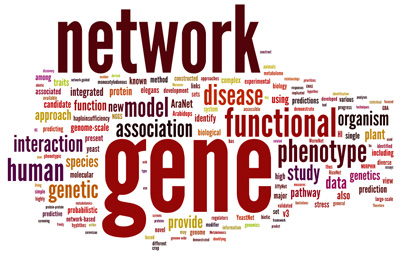
Research Highlight
- 2011 Nature Reviews Genetics, Research highlight (Predicting genetic interaction)
- 2008 Bioessay, What the papers say (WormNet)
- 2008 Genome Biology, Minireview (WormNet)
- 2008 Nature methods, Research highlight (WormNet)
- 2008 Nature Genetics coverstory (WormNet): Network perturbation predicts phenotype
Collaborators
Human/Animal Systems Biology
- Edward Marcotte, University of Texas at Austin, USA
- Ben Lehner, Systems Biology Unit, EMBL-CRG, Spain
- Jonghoon Kim, Korea University, Korea
Crop/Plant Systems Biology
- Sue Rhee, Carnegie Institution of Science, USA
- Pamela Ronald, University of California at Davis, USA
- Thomas Brutnell, Danforth Plant Science Center, USA
- Sangdong Yoo, Korea University, Korea
Microbial Systems Biology
- Yongsun Bahn, Yonsei University, Korea
- Sangsun Yoon, Yonsei Medical School, Korea